NLP(二十五)实现ALBERT+Bi-LSTM+CRF模型
山阴少年 人气:0
在文章[NLP(二十四)利用ALBERT实现命名实体识别](https://blog.csdn.net/jclian91/articlehttps://img.qb5200.com/download-x/details/104806598)中,笔者介绍了ALBERT+Bi-LSTM模型在命名实体识别方面的应用。
在本文中,笔者将介绍如何实现ALBERT+Bi-LSTM+CRF模型,以及在人民日报NER数据集和CLUENER数据集上的表现。
功能项目方面的介绍里面不再多介绍,笔者只介绍模型训练和模型预测部分的代码。项目方面的代码可以参考文章[NLP(二十四)利用ALBERT实现命名实体识别](https://blog.csdn.net/jclian91/articlehttps://img.qb5200.com/download-x/details/104806598),模型为ALBERT+Bi-LSTM+CRF,结构图如下:
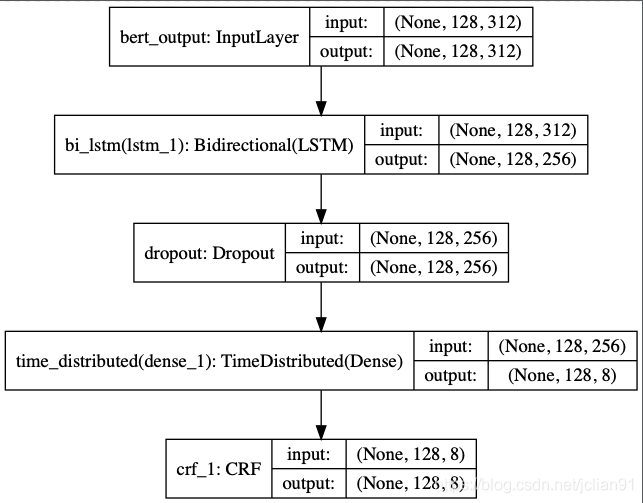
模型训练的代码(albert_model_train.py)中新增导入keras-contrib模块中的CRF层:
```python
from keras_contrib.layers import CRF
from keras_contrib.losses import crf_loss
from keras_contrib.metrics import crf_accuracy, crf_viterbi_accuracy
```
模型方面的代码如下:
```python
# Build model
def build_model(max_para_length, n_tags):
# Bert Embeddings
bert_output = Input(shape=(max_para_length, 312, ), name="bert_output")
# LSTM model
lstm = Bidirectional(LSTM(units=128, return_sequences=True), name="bi_lstm")(bert_output)
drop = Dropout(0.1, name="dropout")(lstm)
dense = TimeDistributed(Dense(n_tags, activation="softmax"), name="time_distributed")(drop)
crf = CRF(n_tags)
out = crf(dense)
model = Model(inputs=bert_output, outputs=out)
# model.compile(optimizer='adam', loss='categorical_crossentropy', metrics=['accuracy'])
model.compile(loss=crf.loss_function, optimizer='adam', metrics=[crf.accuracy])
# 模型结构总结
model.summary()
plot_model(model, to_file="albert_bi_lstm.png", show_shapes=True)
return model
```
设置文本的最大长度MAX_SEQ_LEN = 128,训练10个epoch,在测试集上的F1值(利用seqeval模块评估)输出如下:
```
precision recall f1-score support
LOC 0.9766 0.9032 0.9385 3658
ORG 0.9700 0.9465 0.9581 2185
PER 0.9880 0.9721 0.9800 1864
micro avg 0.9775 0.9321 0.9543 7707
macro avg 0.9775 0.9321 0.9541 7707
```
之前用ALBERT+Bi-LSTM模型得到的F1值为91.96%,而ALBERT+Bi-LSTM+CRF模型能达到95.43%,提升效果不错。
模型预测代码(model_predict.py)如下:
```python
# -*- coding: utf-8 -*-
# author: Jclian91
# place: Pudong Shanghai
# time: 2020-03-11 13:16
import json
import numpy as np
from keras_contrib.layers import CRF
from keras_contrib.losses import crf_loss
from keras_contrib.metrics import crf_accuracy, crf_viterbi_accuracy
from keras.models import load_model
from collections import defaultdict
from pprint import pprint
from utils import MAX_SEQ_LEN, event_type
from albert_zh.extract_feature import BertVector
# 读取label2id字典
with open("%s_label2id.json" % event_type, "r", encoding="utf-8") as h:
label_id_dict = json.loads(h.read())
id_label_dict = {v: k for k, v in label_id_dict.items()}
# 利用ALBERT提取文本特征
bert_model = BertVector(pooling_strategy="NONE", max_seq_len=MAX_SEQ_LEN)
f = lambda text: bert_model.encode([text])["encodes"][0]
# 载入模型
custom_objects = {'CRF': CRF, 'crf_loss': crf_loss, 'crf_viterbi_accuracy': crf_viterbi_accuracy}
ner_model = load_model("%s_ner.h5" % event_type, custom_objects=custom_objects)
# 从预测的标签列表中获取实体
def get_entity(sent, tags_list):
entity_dict = defaultdict(list)
i = 0
for char, tag in zip(sent, tags_list):
if 'B-' in tag:
entity = char
j = i+1
entity_type = tag.split('-')[-1]
while j < min(len(sent), len(tags_list)) and 'I-%s' % entity_type in tags_list[j]:
entity += sent[j]
j += 1
entity_dict[entity_type].append(entity)
i += 1
return dict(entity_dict)
# 输入句子,进行预测
while 1:
# 输入句子
text = input("Please enter an sentence: ").replace(' ', '')
# 利用训练好的模型进行预测
train_x = np.array([f(text)])
y = np.argmax(ner_model.predict(train_x), axis=2)
y = [id_label_dict[_] for _ in y[0] if _]
# 输出预测结果
pprint(get_entity(text, y))
```
在网上找几条新闻,预测结果如下:
```
Please enter an sentence: 驴妈妈旅游网创始人洪清华近日接受媒体采访谈及驴妈妈的发展模式时表示:现在,电商有两种做法——小而美的电商追求盈利,大而全的电商钟情规模。
{'PER': ['洪清华']}
Please enter an sentence: EF英孚教育集团是全球最大的私人英语教育机构,主要致力于英语培训、留学旅游以及英语文化交流等方面。
{'ORG': ['EF英孚教育集团']}
Please enter an sentence: 宋元时期起,在台湾早期开发的过程中,中华文化传统已随着大陆垦民传入台湾。
{'LOC': ['台湾', '中华', '台湾']}
Please enter an sentence: 吸引了众多投资者来津发展,康师傅红烧牛肉面就是于1992年在天津诞生。
{'LOC': ['天津']}
Please enter an sentence: 经过激烈角逐,那英战队成功晋级16强的学员有实力非凡的姚贝娜、挚情感打动观众的朱克、音乐创作能力十分突出的侯磊。
{'PER': ['姚贝娜', '朱克', '侯磊']}
```
接下来我们看看该模型在CLUENER数据集上的表现。CLUENER数据集是在清华大学开源的文本分类数据集THUCTC基础上,选出部分数据进行细粒度命名实体标注,原数据来源于Sina News RSS,实体有:地址(address),书名(book),公司(company),游戏(game),政府(goverment),电影(movie),姓名(name),组织机构(organization),职位(position),景点(scene),该数据集的介绍网站为:[https://www.cluebenchmarks.com/introduce.html](https://www.cluebenchmarks.com/introduce.html) 。
下载数据集,用脚本将其处理成模型支持的数据格式,因为缺少test数据集,故模型评测的时候用dev数据集代替。设置模型的文本最大长度MAX_SEQ_LEN = 128,训练10个epoch,在测试集上的F1值(利用seqeval模块评估)输出如下:
```
sentences length: 10748
last sentence: 艺术家也讨厌画廊的老板,内心恨他们,这样的话,你是在这样的状态下,两年都是一次性合作,甚至两年、
start ALBERT encding
end ALBERT encoding
sentences length: 1343
last sentence: 另外意大利的PlayGeneration杂志也刚刚给出了92%的高分。
start ALBERT encding
end ALBERT encoding
sentences length: 1343
last sentence: 另外意大利的PlayGeneration杂志也刚刚给出了92%的高分。
start ALBERT encding
end ALBERT encoding
......
.......
precision recall f1-score support
book 0.9343 0.8421 0.8858 152
position 0.9549 0.8965 0.9248 425
government 0.9372 0.9180 0.9275 244
game 0.6968 0.6725 0.6844 287
organization 0.8836 0.8605 0.8719 344
company 0.8659 0.7760 0.8184 366
address 0.8394 0.8187 0.8289 364
movie 0.9217 0.7067 0.8000 150
name 0.8771 0.8071 0.8406 451
scene 0.9939 0.8191 0.8981 199
micro avg 0.8817 0.8172 0.8482 2982
macro avg 0.8835 0.8172 0.8482 2982
```
在网上找几条新闻,预测结果如下:
```
Please enter an sentence: 据中山外侨局消息,近日,秘鲁国会议员、祖籍中山市开发区的玛利亚·洪大女士在秘鲁国会大厦亲切会见了中山市人民政府副市长冯煜荣一行,对中山市友好代表团的来访表示热烈的欢迎。
{'address': ['中山市开发区', '秘鲁国会大厦'],
'government': ['中山外侨局', '秘鲁国会', '中山市人民政府'],
'name': ['玛利亚·洪大', '冯煜荣'],
'position': ['议员', '副市长']}
Please enter an sentence: “隔离结束回来,发现公司不见了”,网上的段子,真发生在了昆山达鑫电子有限公司员工身上。
{'company': ['昆山达鑫电子有限公司']}
Please enter an sentence: 由黄子韬、易烊千玺、胡冰卿、王子腾等一众青年演员主演的热血励志剧《热血同行》正在热播中。
{'game': ['《热血同行》'], 'name': ['黄子韬', '易烊千玺', '胡冰卿', '王子腾'], 'position': ['演员']}
Please enter an sentence: 近日,由作家出版社主办的韩作荣《天生我才——李白传》新书发布会在京举行
{'book': ['《天生我才——李白传》'], 'name': ['韩作荣'], 'organization': ['作家出版社']}
```
本项目已经开源,Github网址为:[https://github.com/percent4/ALBERT_NER_KERAS](https://github.com/percent4/ALBERT_NER_KERAS) 。
本文到此结束,感谢大家阅读,欢迎关注笔者的微信公众号:Python爬虫与算法。
加载全部内容